Trial: AI Judgement—Evaluating an AI Arbitrator
In this AI Trial, we assess how generative instruct models perform as judging entities. Human evaluators examine AI's capacity for rational, impartial, and ethical decision-making. The study aims to identify the most effective models and tools for AI-driven arbitration in complex scenarios.
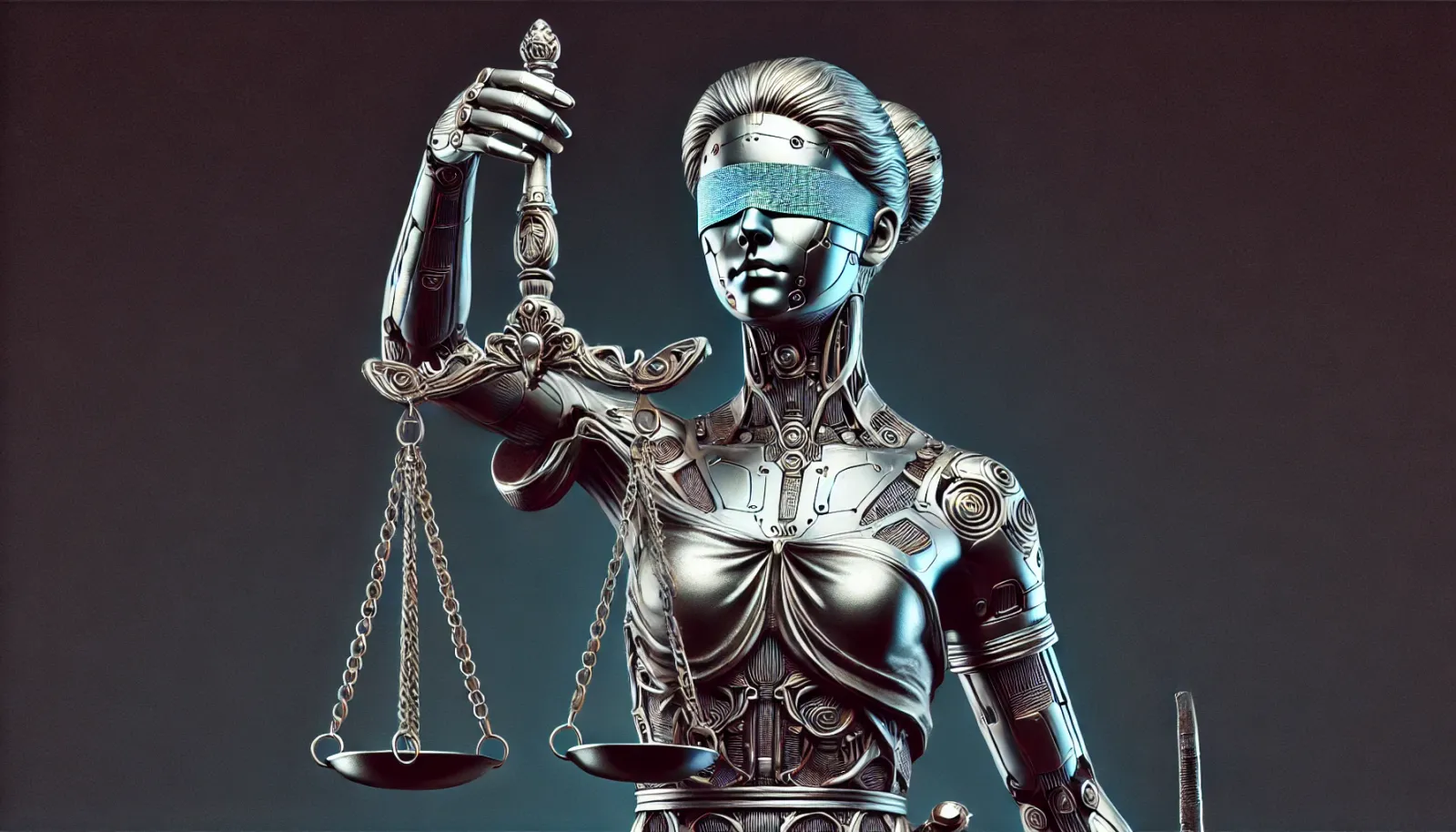
The emergence of generative text models has opened up numerous applications that demand judgment and the ability to process diverse contextual information. Perhaps the most striking characteristic of large language models is their capacity for reasoning. It is surprising to discover that rational thinking is inherently embedded in human language. Upon reflection, one might conclude that advanced mimicry of language patterns may indeed approximate the behavior of rational agents, even when rationality is not explicitly trained but rather acquired through the universal proxy of language itself.
Assessment Criteria
To evaluate the effectiveness of an AI arbitrator, a panel of human judges will assess the AI's performance based on several key criteria:
- Rationality and Logic. The AI must demonstrate a clear, rational chain of thought and provide comprehensive explanations for its decisions.
- Impartiality: The AI arbitrator should maintain objectivity, even when faced with scenarios that could potentially benefit from biased judgments.
- Deterrence and Marginality: An effective AI judge should recognize when differences between options are marginal and be willing to declare a tie rather than make an arbitrary decision.
- Consistency: The AI must apply standards and criteria uniformly across various cases to ensure fairness.
- Ethical Considerations: The ability to identify and address ethical dilemmas is crucial for maintaining the integrity of the judgment process.
- Transparency and Justification: Clear articulation of reasoning behind judgments is essential for accountability and understanding.
This trial aims to determine whether advanced language models can truly approximate rational agents capable of fair and effective arbitration. But most importantly, it seeks to identify which models and tools are best suited for this complex task, paving the way for more reliable and efficient AI-driven arbitration systems.
Nominations and Face-Offs
Completed
- GPT-4o (OpenAI) / GPT-4o mini (OpenAI)
- Command R (Cohere) / Llama 3 70B (Meta)
- Llama 3 8B Instruct / Mixtral 8x7B Instruct
- Claude 3.5 Sonnet / OpenAI o1-preview