Trial: AI-Generated Text Detection
This Field Trial examines tools and techniques for detecting AI-authored content, focusing on accuracy, robustness, and explainability—essential factors in maintaining content quality in the digital age.
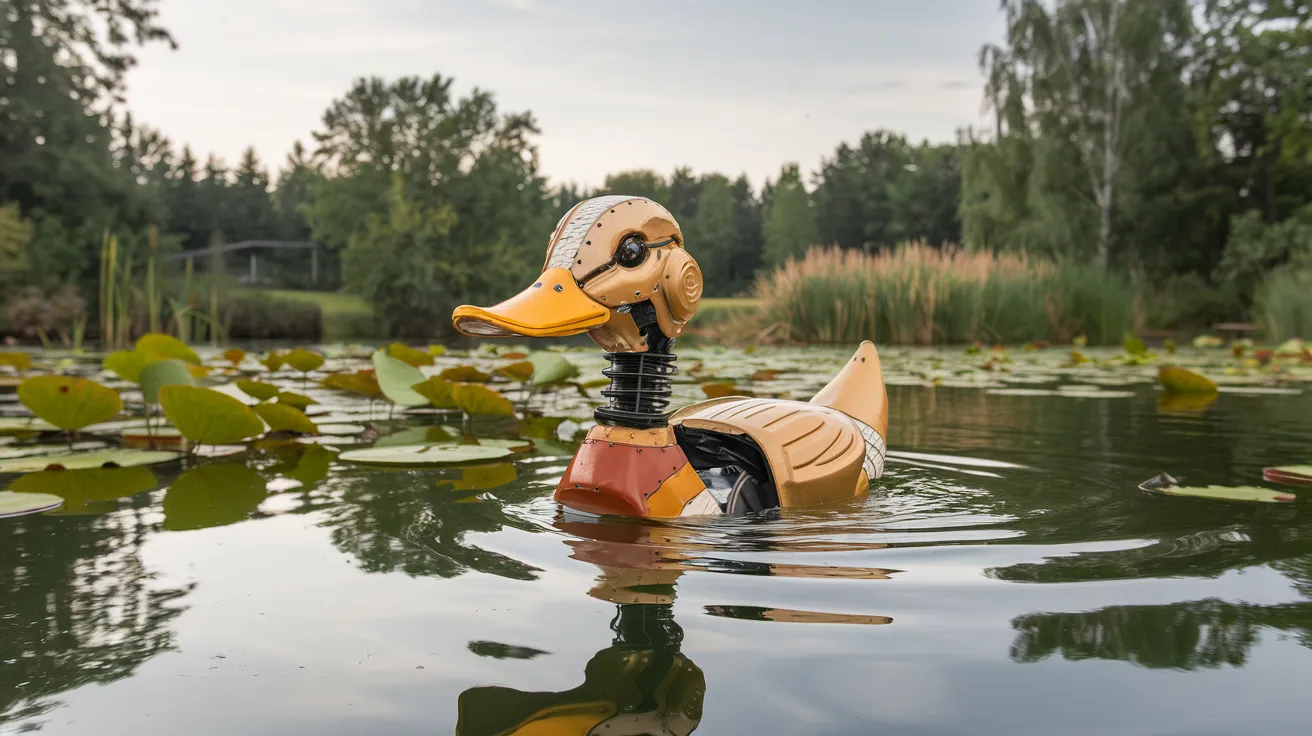
In a world where AI tools for content generation are easily accessible, the volume of produced information leads to a surge. While these efficient tools save creators' time or increase output volume, the quality of the content may suffer if rushed or not given enough thought, editing, or review. More particularly, it became apparent that many consumer markets nowadays use generative AI as spokesmen, prevailing in marketing and sales.
In this trial, we'll assess tools, workflows, and techniques for detecting AI-generated text content. Such tools are valuable for validating high-quality content and avoiding artificial tones in writing.
If it looks like a duck, swims like a duck, and quacks like a duck, then it probably is a duck ¹
Assessment Criteria
First, we'll assess the tools' accuracy to identify whether the content is human or AI-generated. The assessment dataset is balanced with roughly a 50% split. We'll measure the frequency of mislabelling human-written content as AI-generated and vice-versa. Finally, we'll use the F1-score, a balanced measure of precision and recall.
Part of the assessment is robustness across different content types, like articles, papers, social media posts, marketing copy, etc. The dataset contains a variety of written and generated content, including mixing generation and human editing.
Finally, we'll assess the explainability of each tool to make sure these tools transparently report the detection methods, provision reasoning or confidence scores for classifications, or highlight specific parts of the text that influenced the decision.
¹ Duck Test, which aptly applies to identifying AI-generated content.